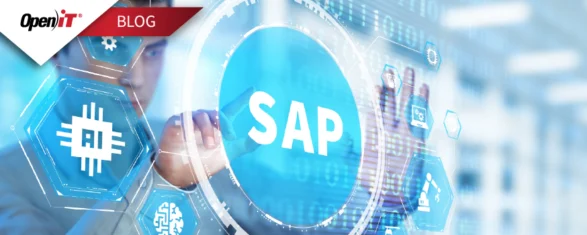
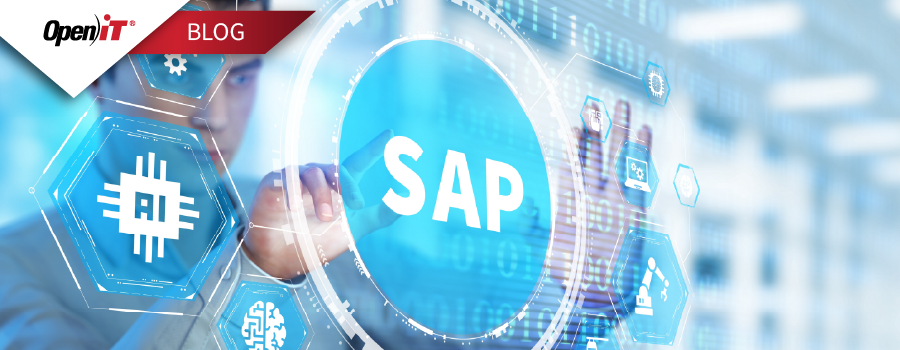
SAP S/4HANA Migration: Are You There Yet?
Despite nearly a decade since the launch of SAP S/4HANA, only 34% of organizations have fully migrated as of early
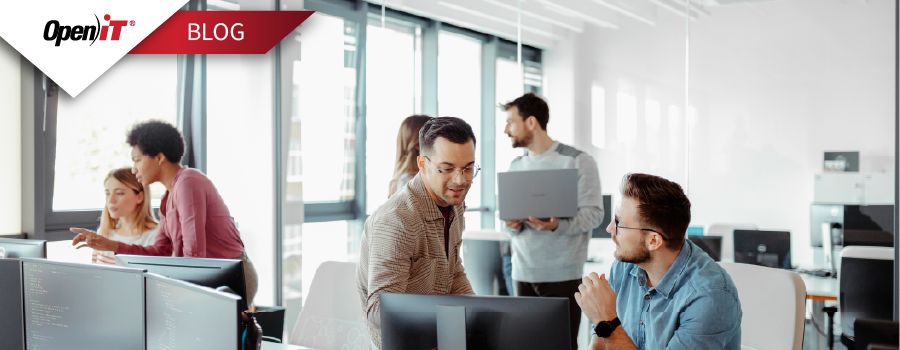
Siemens and Altair: Post-Acquisition License Implications for Federal Agencies
Siemens’ recent acquisition of Altair expands its digital engineering footprint across disciplines including MBSE, PLM, HPC, and data analytics. For
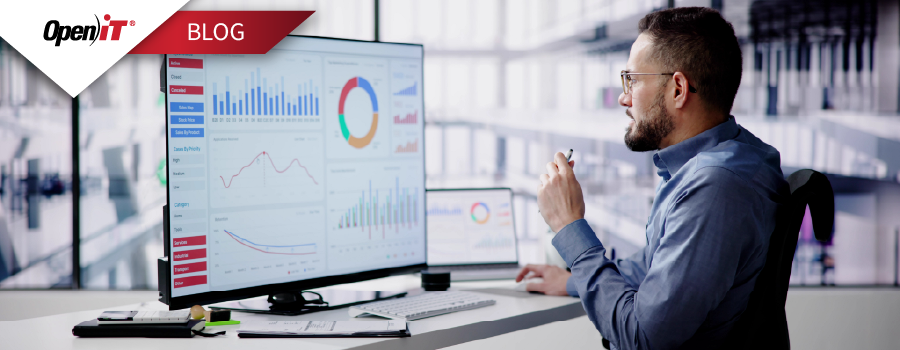
Open iT and Bluebeam®: Optimize Licenses Based on Actual Usage
What Could Bluebeam Licensing Optimization Look Like? Picture this: a global firm is preparing to shift from Bluebeam®’s legacy concurrent
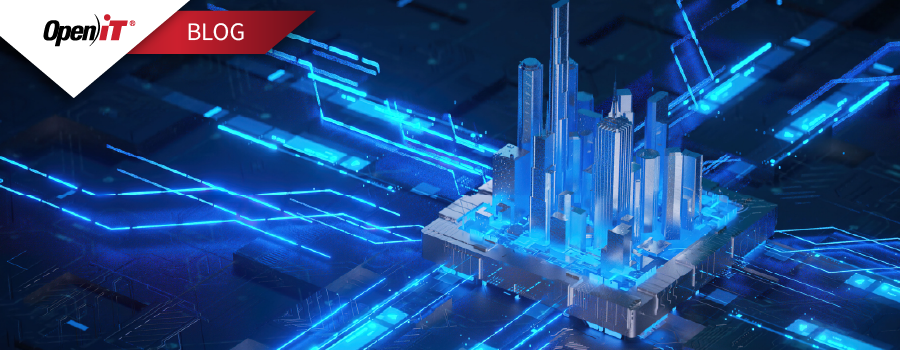
AEC Software: A Strategic Lever for Federal Infrastructure Resilience
With more than $1.2 trillion allocated through the Infrastructure Investment and Jobs Act (IIJA), federal agencies face mounting pressure to
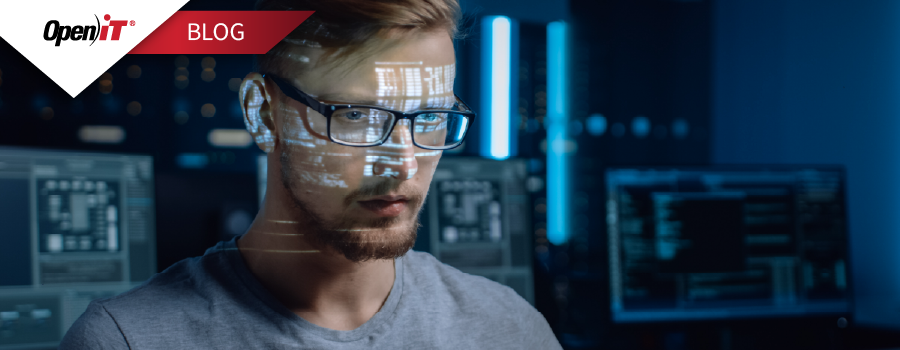
Transformational CIOs: Empowering Modern IT Leaders with License Usage Data
In engineering-driven sectors—manufacturing, automotive, aerospace, defense, and architecture, engineering, and construction (AEC)—the role of the CIO is undergoing a fundamental
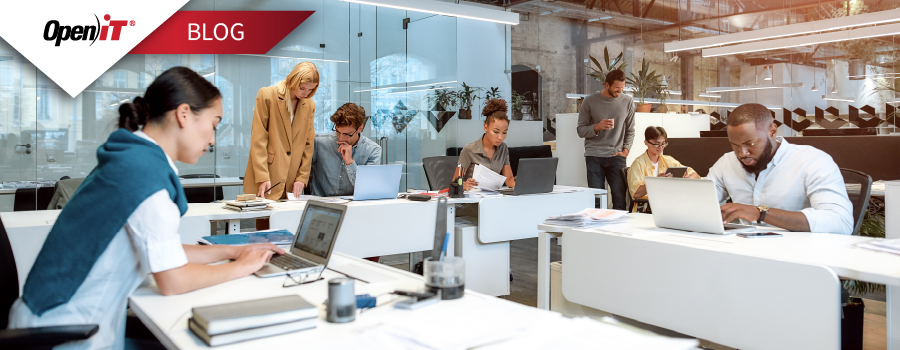
LicenseAnalyzer™ Level 3: Automated License Harvesting for Maximum Efficiency
Part 3 of 3 in the LicenseAnalyzer™ Series LicenseAnalyzer™ Level 3 delivers automated license reclamation to reduce waste, improve software
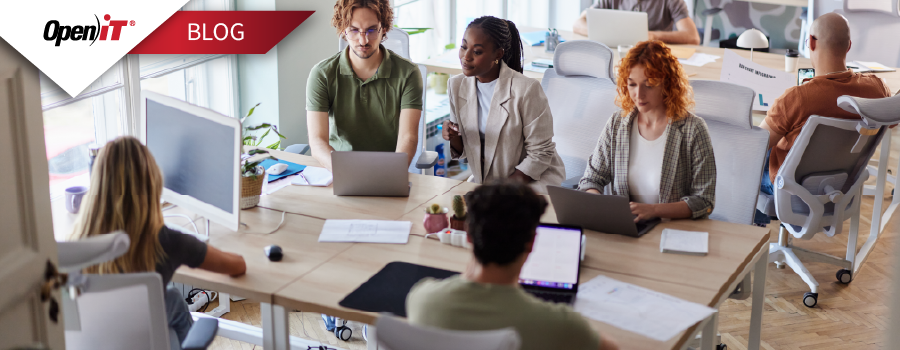
Duplicate Federal Software Purchases: A GAO-Estimated $100B License Optimization Opportunity
The Government Accountability Office (GAO) estimates that that federal agencies could save at least $100 billion in federal IT Spending
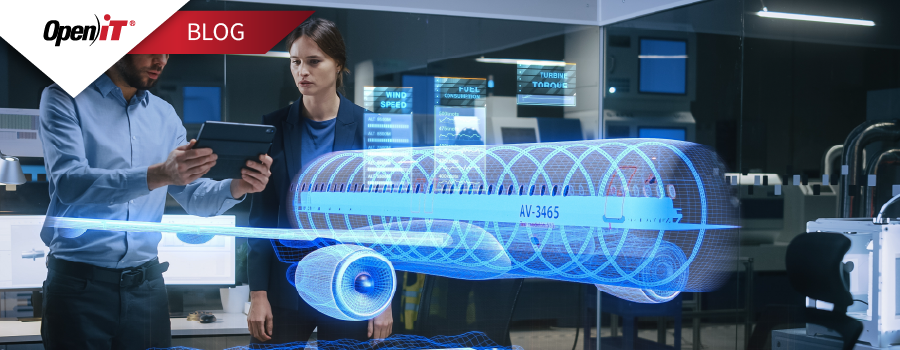
Rethink Software Cost Estimation in Aerospace & Defense
As of 2024, the Aerospace & Defense industry (A&D) invests approximately $7.7 billion a year in specialized software, and market
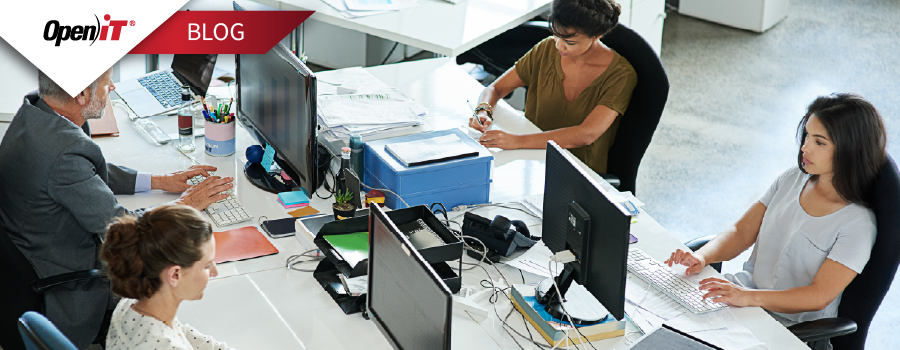
Open iT LicenseAnalyzer™ Level 2: Accountability Through True Active Usage
Part 2 of 3 in the LicenseAnalyzer™ Series Move beyond visibility. Gain a clear picture of how, where, and by whom